Table of Contents
What is Data Science?
Definition One:
Data science proceeds to emerge as one of the various encouraging and in-demand professional pathways for experienced specialists. Presently, flourishing data specialists understand that people need to develop beyond some universal talents of investigating massive quantities of data, data mining, and programming skills. To reveal valuable knowledge for their businesses, data experts need to master the entire spectrum of the data science development circle and maintain a level of adaptability.
Definition Two:
data science course is an interdisciplinary course that utilizes experimental techniques, methods, algorithms, and methods to obtain benefits from data. Data scientists consolidate a variety of skills—including statistics, network science, and industry experience—to interpret data secured from the interconnection, smartphones, clients, sensors, including other references. Data science exposes courses and provides penetrations that manufacturers can practice to obtain better choices and generate more innovative products and services. Data is the bedrock of variation, but its significance originates from the knowledge data scientists can discover from it and then act simultaneously.
Definition Three:
Data science is an interdisciplinary course that applies innovative methods, manners, algorithms, and methods to obtain information and insights of various organized and unorganized data. Data science is said to be data processing, deep learning, and large data. Data science may be a “concept to unify statistics, data analysis, machine learning, domain knowledge, and their related methods” to “understand and analyze actual phenomena” with data. It uses techniques and theories drawn from many fields within the context of mathematics, statistics, computing, domain knowledge, and knowledge science. Turing grant victor Jim Gray perceived data science as essentially a “fourth model” of science and stated that “everything concerning science is evolving recognition to the influence of information technology” and the data deluge.
The rapid spread and global impacts of COVID-19 can make people feel helpless and scared as the novel coronavirus escalates and forces them to change many aspects of their everyday lives.
However, people can feel glimmers of hope in these uncertain times by understanding more about how data scientists are working hard to learn as much about COVID-19 as they can.
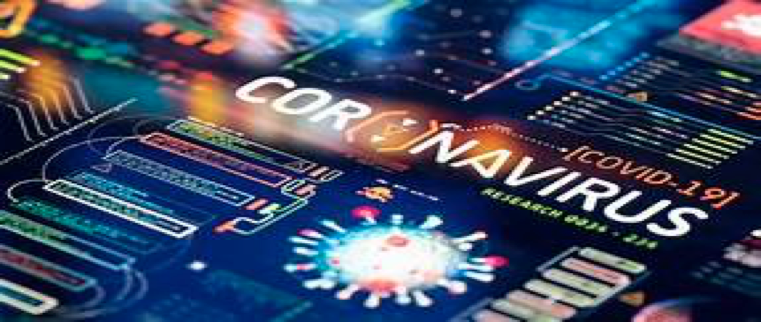
Data Science Can Give Accurate Pictures of Coronavirus Outcomes:
Medical professionals and others must get correct and up-to-date information about how the coronavirus situation changes day by day. Several organizations, including Johns Hopkins University, IBM, and Tableau, have released interactive databases that offer real-time views of what’s happening with the virus.
Many of these sources pull from data provided by trusted bodies such as the U.S Centers for Disease Control and Prevention (CDC) and the World Health Organization (WHO). They also include direct links to those places so that people have quick, easy access to reliable information.
Using these databases can inform people of the number of confirmed cases, fatalities, and recoveries. Then, whether a person is on the front lines of the coronavirus fight or a concerned citizen trying to stay informed, they can get all or most of the information they need in one place.
Looking forward to becoming a Data Scientist? Check out the Data Science Bootcamp Program and get certified today.
Data Scientists Devise a Speedier Way to Handle Contact Tracing:
Contact tracing is an effective way to slow COVID-19. It involves getting in touch with a person’s close contacts after that individual tests positive for the virus and telling them to self-isolate. Contact tracing is time-consuming, although it’s getting easier as more people take social distancing seriously.
Data scientists and medical experts teamed up at Oxford University to make contact tracing even more efficient. The experts working on the project asserted that mathematical models showed them how traditional methods of contact tracing used in public health are not fast enough to thoroughly slow the spread of COVID-19.
They created a mobile phone-based solution to eliminate the need for people to call the contacts manually. Instead, those parties get text messages confirming the need for self-isolation. The researchers clarify that their approach would be most effective if it gets support from national leaders and is not an effort primarily spearheaded by independent app developers.
No nations are using this method yet. Given the market penetration of mobile phones and the familiarity people have with receiving texts, however, it’s easy to see why this approach makes sense.
Everyone Can Play a Part in Helping Scientists Fight the Coronavirus
Many people with COVID-19 have only mild symptoms or none at all. Plus, the classic symptoms include a fever and a cough — two issues not restricted to the coronavirus. These things could make it easier for people to unknowingly spread the disease. But, developers created an app that uses data-sharing to help medical experts learn more about the virus.
It’s called the COVID Symptom Tracker and already has at least 200,000 users. People can and should interact with the app even if they are asymptomatic or do not think their symptoms are COVID-19-related. The more researchers know about the coronavirus, the better equipped they are to tackle it.
People interact with the app to do a short daily symptom check-in. They also give their age and zip code, plus disclose any preexisting conditions. That information helps scientists determine the groups that are most affected or in danger. The app does not take user data for commercial purposes, but it gives it to people who are working to stop the coronavirus, including some at health organizations.
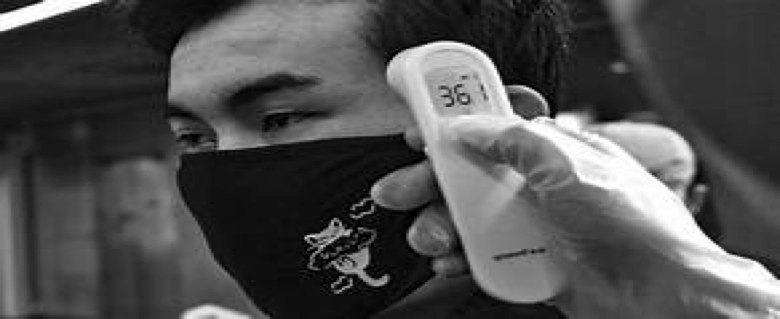
Data Scientists Use Machine Learning to Find Possible Cures Faster:
Besides the race to restrict the COVID-19 spread, scientists are working as quickly as possible to uncover effective treatments. Two graduates of the data science program at Columbia University have turned to machine learning to help. The typical process of antibody discovery during a lab takes years. This approach, however, takes only every week to screen for therapeutic antibodies with a high likelihood of success.
The team taking this approach says this method is less costly than traditional ones, too. Humans are still part of the process because they have to test the gene sequences identified as most promising by the machine learning algorithm. However, using this expedited method could be crucial in efficiently finding interventions that work for coronavirus patients.
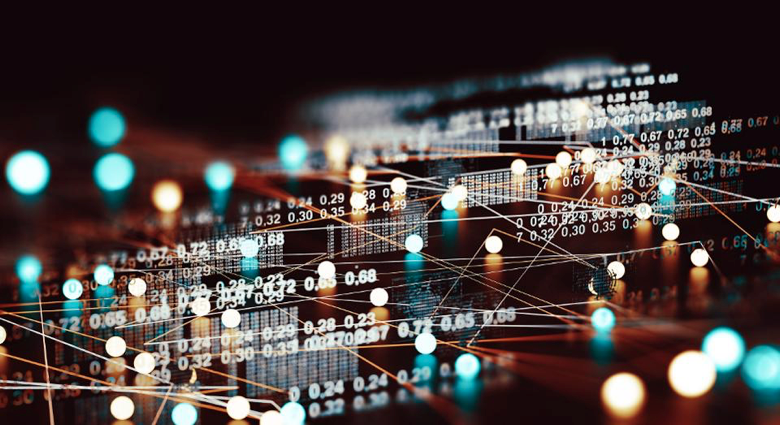
Data Science Can Help Track the Spread:
Data science specialists have also concluded that graph databases are instrumental in showing them how COVID-19 spreads. Each plan database explains the connections between personalities, situations, or objects. Scientists refer to each of those entities as a node, and the connections between them are the “edges.” The results give a visual representation of the relationship between things if any.
In the youth of the coronavirus outbreak, Chinese data scientists built a graph database tool called Epidemic Spread. It allowed people to type in identifying information associated with the journeys they took, such as a flight number or even a car’s license plate. The database would then tell those users whether anyone with a confirmed coronavirus case took those same trips and may have spread it to fellow passengers.
Making Progress Against COVID-19
Knowing as much about the coronavirus as possible will save lives. These are only some of the fascinating ways that data scientists are using their skills to help.
Open Source Data Science on Fight COVID-19 (Corona Virus):
With the spread of COVID-19 becoming an ever more assertive force in our lives, the healthcare data science community has an opportunity to play an important role in the mitigation of this emerging pandemic. Chronicle has given acknowledgment to the before-mentioned complications vessel drastically change those most harmful consequences of the before-mentioned infections. Many cities have imposed social distancing measures, closing any place where large numbers of people gather, and further measures can be taken to help isolate and protect the most vulnerable among the population. To do so, we must first identify who is at greatest risk, which motivated my team to create an open-sourced project, the COVID-19 vulnerability Index. This COVID-19 Dictionary is an open-source, AI-based imminent design that distinguishes personalities that do anticipate maintaining a heightened vulnerability before the critical complexities of COVID-19.
The COVID-19 Index is meant to assist hospitals, federal / state / local public health agencies, and other healthcare organizations in their work to spot, plan for, answer, and reduce the impact of COVID-19 in their communities. In this post, we’ll be going over the high-level details of this open-sourced project.
Detailed Description of Data Selection:
Step 1:
Making a Labeled Data Set Data on COVID-19 hospitalizations do not yet exist. While data begins to emerge, we can look at the affected populations and events that serve as proxies for the real event. Given that the disease’s worst outcomes are concentrated on the elderly, we can focus on medicare billing data. Instead of predicting COVID-19 hospitalizations, we can instead predict proxy medical events, specifically hospitalizations due to respiratory infections. Examples include Pneumonia, Influenza, and Acute bronchitis. We identify these labels by parsing medical billing data and searching for specific ICD-10 codes that describe these types of events. All predictions are made on a specific day. From a particular day, we look back in time 15 months for features. We exclude any events happening within three months of the prediction date, due to the lag in medical claims data reporting. Any diagnoses within the last year become the features we use in all of our models.
Step 2 :
Models There are hosts of model considerations that need to be made with these kinds of projects. Ultimately, we wanted these models to balance being as effective as possible, and still accessible to healthcare data scientists as quickly as possible. One of the reasons for choosing the data that we used in because medicare claims data is widely available to healthcare data scientists. If your organization has access to additional data sources, you may observe performance increases by incorporating such information. Balancing those considerations led us to create 3 models based on the ease of adoption and model effectiveness. The first is a logistic regression model using a small number of features. At Closed Loop, we use the quality Python data science stack.
The motivation for a very simple model is that it can be ported to environments like R or SAS without having to read or write a line of python. At low alert rates, the model performs close to parity with the more sophisticated versions of the model. The aforementioned white paper has all of the weights for the limited feature set, so it can be ported over by hand. ROC graph comparing the performance of all three models. The next two models are both made using XGBoost. XGBoost consistently gives the best performance for making predictions on well-structured data, and given the right data transformation, medical billing data has that structure. The first XGBoost model is featured in our open-sourced package. A pickled version of the model exists, so you simply need to build a data transformation pipeline that will get your billing data into the format specified in the repo.
If you can build a function that will parse your data for a specific code, then you can simply iterate through all of the codes. That’s the reason we selected a limited feature set for the open-sourced model. It’s very effective, while still requiring only a reasonable level of lift from the data pipeline standpoint. We’re also giving healthcare organizations access to our model within the platform. This version of the model uses full diagnosis history, plus a large set of engineered features. Perceive, the ROC slider determines that the open-source report becomes an approximately alike appearance as the report included in our program
Error 404: Data Not Found!
Have it in the bag? Data (or its lack thereof) can be the biggest and most overlooked challenge when it comes to the adoption of data science. Many organizations don’t have the necessary data to perform data science. Legacy practices, common examples of which include – data captured through physical forms, unstructured data, no scalable IT infrastructure in place to process data, and data stored in remote silos, are the primary reason that some organizations are not even aware that the data they have is of no practical use. Prioritizing data collection and digitization of data from existing sources is the frontline solution to this problem. However, it is also important for companies to explore new data sources while enhancing data accessibility for all key stakeholders.
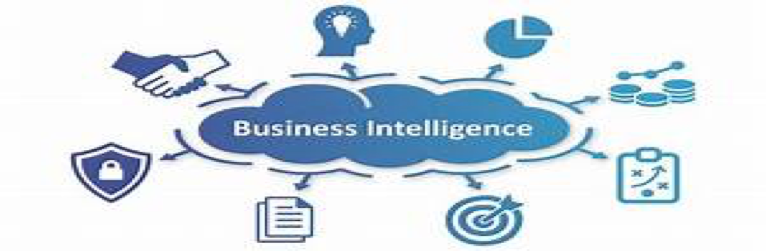
What Is Business Intelligence – BI?
Business intelligence (BI) refers to the procedural and technical support that handles, buildings, and interprets the data provided by a company’s actions. Business intelligence (BI) is a broad term that encompasses data mining, process analysis, performance benchmarking, and descriptive analytics. Business intelligence (BI) parses all the data generated by a business and presents easy-to-digest reports, performance measures, and trends that inform management decisions. Origins of Business intelligence (BI) The need for Business intelligence (BI) was derived from the concept that managers with inaccurate or incomplete information will tend, on average, to make worse decisions than if they had better information. Creators of financial models recognize this as “garbage in, garbage out.” Business intelligence (BI) attempts to solve this problem by analyzing current data that is ideally presented on a dashboard of quick metrics designed to support better decisions. Most companies can benefit from incorporating Business intelligence (BI) solutions; managers with inaccurate or incomplete information will tend, on average, to make worse decisions than if they had better information. The Growing Field To obtain helpfully, Business intelligence (BI) needs to attempt to improve the efficiency, opportunity, and significance of data. These requirements mean finding more ways to capture information that is not already being recorded, checking the information for errors, and structuring the information in a way that makes broad analysis possible. In practice, however, companies have data that is unstructured or in diverse formats that do not make for easy collection and analysis. Software firms thus provide business intelligence solutions to optimize the information gleaned from data. These are enterprise-level software administrations intended to join a company’s information including analytics. Although software solutions continue to evolve and are becoming increasingly sophisticated, there is still a need for data scientists to manage the trade-offs between speed and the depth of reporting. Some of the insights emerging from big data have companies scrambling to capture everything, but data analysts can usually filter out sources to find a selection of data points that can represent the health of a process or business area as an entire. This can reduce the necessity to capture and reformat everything for analysis, which saves analytical time and increases the reporting speed.
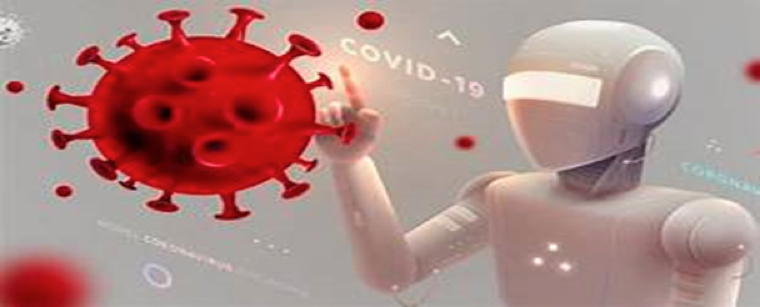
India: Rethinking Digitization And Data Science In COVID-19 World:
You might have come across this cliché – COVID-19 has accelerated the shift to digital. With ongoing lockdowns and a projected recession, businesses are struggling to keep up with day-to-day operations and making tough decisions like layoffs, salary cuts, and Capex rollbacks. While the present seems bleak and the future looks uncertain, businesses find themselves amidst an unprecedented crisis with only one thing certain: The future is digital. While some industries quickly adapted to remote work and digital tools, others had to deal with multiple challenges to maintain business continuity. Business leaders have been busy with the adaptation of new operating models, optimizing business processes, measuring RoI of various spending, and gauging long-term business impact through data science and data-driven scenario simulations.
Governments and healthcare providers worldwide have adopted data science in mitigating the impact of COVID-19:
This has been possible through the digital tracking of patients to monitor disease spread through epidemic forecast models to allocate healthcare resources through molecular modeling in drug and vaccine discovery and more. Access to quality data and data science experts to apply enhanced techniques is proving to be critical for faster recovery. With no travel and reduced meeting hours, it could be an apt time for CXOs to rethink their future in this changing business environment.
Data and data science are two key ingredients of any digital operating model:
While data science might seem like a luxury today amidst this struggle for survival, it could be a differentiating factor in deciding winners of tomorrow. With a few visionary companies already ahead on the curve, all organizations must plan their digital strategy to adapt to the post-COVID normal before it looks us in the eye. In the same context, this article discusses the potential roadblocks in the adoption of data science and possible ways to sidestep them. Culture Conundrums The storming of the Bastille! Yes, this is where we get to complain about corporate culture.
Gut-based decision-making, multitudes of excel reports, never-ending budget forecasts, and out-of-the-world sales targets! But things could be better if all decisions were backed by data and facts so that everyone could see the underlying rationale while supporting and contributing to the decision-making process. Undoubtedly, commitment from the top leadership is vital. A top-down mandate alone can’t ensure the wide use of data science for decision-making throughout the business. A bottom-up adoption to embed data science into the way the organization thinks, decides, and acts are necessary for good results.
Coming to End:
The whole world is participating in a fight against this pandemic. The healthcare data science community can have a big impact on combating this disease. There have been many excellent efforts to use data visualization and Carlo simulations to help combat the spread of this pandemic. We feel our model addresses a complementary and important aspect of health policy, identifying those most at risk. By combining the efforts of these and many other excellent efforts in the healthcare technology space, we hope to mitigate the effects of this terrible disease. If reading this article has given you ideas for ways in which you’d like to contribute, we encourage you to be locked.
Recommended Read:
- Top 15 Best Data Science Course in Mumbai
- Top 10 Data Science Course in Pune
- Top 10 Data Science Course in Bangalore
- Top 10 Data Science Courses in Nagpur
- Top 20 Data science course in Delhi NCR
- Top 10 Data Science Course In India
Also Check this Video
Recommended Programs
Data Science Course
With Training
The Data Science Course from Henry Harvin equips students and Data Analysts with the most essential skills needed to apply data science in any number of real-world contexts. It blends theory, computation, and application in a most easy-to-understand and practical way.
Artificial Intelligence Certification
With Training
Become a skilled AI Expert | Master the most demanding tech-dexterity | Accelerate your career with trending certification course | Develop skills in AI & ML technologies.
Certified Industry 4.0 Specialist
Certification Course
Introduced by German Government | Industry 4.0 is the revolution in Industrial Manufacturing | Powered by Robotics, Artificial Intelligence, and CPS | Suitable for Aspirants from all backgrounds
RPA using UiPath With
Training & Certification
No. 2 Ranked RPA using UI Path Course in India | Trained 6,520+ Participants | Learn to implement RPA solutions in your organization | Master RPA key concepts for designing processes and performing complex image and text automation
Certified Machine Learning
Practitioner (CMLP)
No. 1 Ranked Machine Learning Practitioner Course in India | Trained 4,535+ Participants | Get Exposure to 10+ projects
Explore Popular CategoryRecommended videos for you
Learn Data Science Full Course
Python for Data Science Full Course
What Is Artificial Intelligence ?
Demo Video For Artificial intelligence
Introduction | Industry 4.0 Full Course
Introduction | Industry 4.0 Full Course
Demo Session for RPA using UiPath Course
Feasibility Assessment | Best RPA Using Ui Path Online Course